A COMPARATIVE STUDY OF FORECASTING MODELS FOR TREND AND SEASONAL TIME SERIES DOES COMPLEX MODEL ALWAYS YIELD BETTER FORECAST THAN SIMPLE MODELS
Abstract: Many business and
economic time series are non-stationary time series that contain trend and
seasonal variations. Seasonality is a periodic and recurrent pattern caused by
factors such as weather, holidays, or repeating promotions. A stochastic trend
is often accompanied with the seasonal variations and can have a significant
impact on various forecasting methods. In this paper, we will investigate and
compare some forecasting methods for modeling time series with both trend and
seasonal patterns. These methods are Winter's, Decomposition, Time Series
Regression, ARIMA and Neural Networks models. In this empirical research, we
study on the effectiveness of the forecasting performance, particularly to
answer whether a complex method always give a better forecast than a simpler
method. We use a real data, that is airline passenger data. The result shows
that the more complex model does not always yield a better result than a
simpler one. Additionally, we also find the possibility to do further research
especially the use of hybrid model by combining some forecasting method to get
better forecast, for example combination between decomposition (as data
preprocessing) and neural network model.
Keywords: Trend, seasonality,
time series, Neural Networks, ARIMA, Decomposition, Winter's, hybrid model
Penulis: Suhartono
Kode Jurnal: jptindustrigg050008
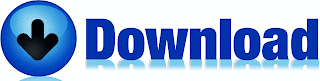