BOT SPAMMER DETECTION IN TWITTER USING TWEET SIMILARITY AND TIME INTERVAL ENTROPY
Abstract: The popularity of
Twitter has attracted spammers to disseminate large amount of spam messages.
Preliminary studies had shown that most spam messages were produced
automatically by bot. Therefore bot spammer detection can reduce the number of
spam messages in Twitter significantly. However, to the best of our knowledge,
few researches have focused in detecting Twitter bot spammer. Thus, this paper
proposes a novel approach to differentiate between bot spammer and legitimate
user accounts using time interval entropy and tweet similarity. Timestamp
collections are utilized to calculate the time interval entropy of each user.
Uni-gram matching-based similarity will be used to calculate tweet similarity.
Datasets are crawled from Twitter containing both normal and spammer accounts.
Experimental results showed that legitimate user may exhibit regular behavior
in posting tweet as bot spammer. Several legitimate users are also detected to
post similar tweets. Therefore it is less optimal to detect bot spammer using
one of those features only. However, combination of both features gives better
classification result. Precision, recall, and f-measure of the proposed method
reached 85,71%, 94,74% and 90% respectively. It outperforms precision, recall,
and f-measure of method which only uses either time interval entropy or tweet
similarity.
Author: Rizal Setya Perdana,
Tri Hadiah Muliawati, Reddy Alexandro
Journal Code: jptkomputergg150004
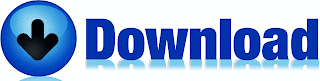
Artikel Terkait :
Jp Teknik Komputer gg 2015
- Current Ripple Analysis of New Double-Stator AC Drive Systems
- Comparing Performances of Evolutionary Algorithms on the Emission Dispatch and Economic Dispatch Problem
- A Polynomial-based Pairwise Key Pre-distribution and Node Authentication Protocol for Wireless Sensor Networks
- Power Characteristics of Wireless Charging for Sensor Networks in Tunnels
- Influence of Sensor Nodes on the Invulnerability of Tree Network
- An Automatic Calibration Method for Near-infrared Camera in Optical Surgical Navigation
- Optimal Economic Ordering Policy with Trade Credit and Discount Cash-Flow Approach
- Design and Implementation of Network Public Opinion Analysis System
- Evaluation of the Modernization of Hydraulic Projects Management Compact-Center-Point Triangular Whitenization Weight Function
- Transmission of Real-time Video Signal with Interference Density and Human Traffic
- Correlation of Student’s Precursor Emotion towards Learning Science Interest using EEG
- The Analysis of Rank Fusion Techniques to Improve Query Relevance
- Recognition of Emotions in Video Clips: The Self-Assessment Manikin Validation
- Automatically generation and evaluation of Stop words list for Chinese Patents
- Service Engineering Based on Service Oriented Architecture Methodology
- Modelling on Tracking Test Condition of Polymer Nanocomposite using Finite Element Simulation
- A Novel Approach to Optimize Cognitive Radio Network Utilization using Cascading Technique
- Classification of Non-Functional Requirements Using Semantic-FSKNN Based ISO/IEC 9126
- Poisson Clustering Process on Hotspot in Peatland Area in Sumatera
- Computing Game and Learning State in Serious Game for Learning
- Double Difference Motion Detection and Its Application for Madura Batik Virtual Fitting Room
- Alternative Technique Reducing Complexity of Maximum Attribute Relation
- High Performance Computing on Cluster and Multicore Architecture
- Control System for Nutrient Solution of Nutrient Film Technique Using Fuzzy Logic
- Measurement of Ultra Wideband Channel Sounding Using Vector Network Analyzer