FEATURE SELECTION METHODS BASED ON MUTUAL INFORMATION FOR CLASSIFYING HETEROGENEOUS FEATURES
Abstract: Datasets with
heterogeneous features can affect feature selection results that are not
appropriate because it is difficult to evaluate heterogeneous features
concurrently. Feature transformation (FT) is another way to handle
heterogeneous features subset selection. The results of transformation from
non-numerical into numerical features may produce redundancy to the original numerical
features. In this paper, we propose a method to select feature subset based on
mutual information (MI) for classifying heterogeneous features. We use
unsupervised feature transformation (UFT) methods and joint mutual information
maximation (JMIM) methods. UFT methods is used to transform non-numerical
features into numerical features. JMIM methods is used to select feature subset
with a consideration of the class label. The transformed and the original
features are combined entirely, then determine features subset by using JMIM
methods, and classify them using support vector machine (SVM) algorithm. The
classification accuracy are measured for any number of selected feature subset
and compared between UFT-JMIM methods and Dummy-JMIM methods. The average
classification accuracy for all experiments in this study that can be achieved
by UFT-JMIM methods is about 84.47% and Dummy-JMIM methods is about 84.24%.
This result shows that UFT-JMIM methods can minimize information loss between
transformed and original features, and select feature subset to avoid redundant
and irrelevant features.
Keywords: Feature selection,
Heterogeneous features, Joint mutual information maximation, Support vector
machine, Unsupervised feature transformation
Author: Ratri Enggar Pawening,
Tio Darmawan, Rizqa Raaiqa Bintana, Agus Zainal Arifin, Darlis Herumurti
Journal Code: jptkomputergg160013
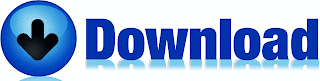