MACULAR EDEMA CLASSIFICATION USING SELF-ORGANIZING MAP AND GENERALIZED LEARNING VECTOR QUANTIZATION
Abstract: Macular edema is a
kind of human sight disease as a result of advanced stage of diabetic retinopathy.
It affects the central vision of patients and in severe cases lead to
blindness. However, it is still difficult to diagnose the grade of macular
edema quickly and accurately even by the medical doctor's skill. This paper
proposes a new method to classify fundus images of diabetics by combining
Self-Organizing Maps (SOM) and Generalized Vector Quantization (GLVQ) that will
produce optimal weight in grading macular edema disease class. The proposed
method consists of two learning phases. In the first phase, SOM is used to
obtain the optimal weight based on dataset and random weight input. The second
phase, GLVQ is used as main method to train data based on optimal weight gained
from SOM. Final weights from GLVQ are used in fundus image classification.
Experimental result shows that the proposed method is good for classification,
with accuracy, sensitivity, and specificity at 80%, 100%, and 60%,
respectively.
Author: Rizal Adi Saputra,
Yuwanda Purnamasari Pasrun, Amaliya Nurani Basyarah
Journal Code: jptkomputergg140010
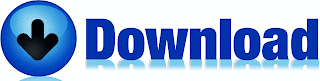