MODEL SELECTION OF ENSEMBLE FORECASTING USING WEIGHTED SIMILARITY OF TIME SERIES
Abstract: Several methods have
been proposed to combine the forecasting results into single forecast namely
the simple averaging, weighted average on validation performance, or
non-parametric combination schemas. These methods use fixed combination of
individual forecast to get the final forecast result. In this paper, quite
different approach is employed to select the forecasting methods, in which
every point to forecast is calculated by using the best methods used by similar
training dataset. Thus, the selected methods may differ at each point to
forecast. The similarity measures used to compare the time series for testing
and validation are Euclidean and Dynamic Time Warping (DTW), where each point
to compare is weighted according to its recentness. The dataset used in the
experiment is the time series data designated for NN3 Competition and time
series generated from the frequency of USPTO’s patents and PubMed’s
scientific publications on the field of health, namely on Apnea, Arrhythmia,
and Sleep Stages. The experimental result shows that the weighted combination
of methods selected based on the similarity between training and testing data
may perform better compared to either the unweighted combination of methods
selected based on the similarity measure or the fixed combination of best
individual forecast.
Keywords: ensemble
forecasting; kesamaan tertimbang; model selection; perkiraan ansambel; seleksi
model; time series; weighted similarity
Author: Agus Widodo, Indra
Budi
Journal Code: jptkomputergg120010
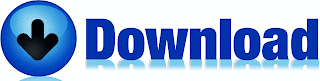
Artikel Terkait :
Jp Teknik Komputer gg 2012
- Fuzzy Expert System for Tropical Infectious Disease by Certainty Factor
- Image Encryption on Mobile Phone using Super Encryption Algorithm
- Securing E-Mail Communication Using Hybrid Cryptosystem on Android-based Mobile Devices
- Particle Filter with Gaussian Weighting for Human Tracking
- View Synthesizing for a Large-Scale Object in a Scene
- Colour Perception on Facial Expression towards Emotion
- Face Recognition Using Holistic Features and Linear Discriminant Analysis Simplification
- Effects of Puncturing Patterns on Punctured Convolutional Codes
- Early Model of Traffic Sign Reminder Based on Neural Network
- Finite Element Method Using PDETOOL of Matlab for Hybrid Stepper Motor Design
- A Low Cost C8051F006 SoC-Based Quasi-Static C-V Meter for Characterizing Semiconductor Devices
- Measurement and Simulation Techniques for Piezoresistive Microcantilever Biosensor Applications
- Optimum Design of a Five-phase Permanent Magnet Synchronous Motor for Underwater Vehicles by use of Particle Swarm Optimization
- Fluctuations Mitigation of Variable Speed Wind Turbine through Optimized Centralized Controller
- Optimal Design of Fuel-cell, Wind and Micro-hydro Hybrid System using Genetic Algorithm
- Optimal Placement and Sizing of Thyristor-controlled-series-capacitor using Gravitational Search Algorithm
- Improved Bacterial Foraging Algorithm for Optimum Economic Emission Dispatch with Wind Power
- Implementation of Electricity Competition Framework with Economic Dispatch Direct Method
- Accelerated Aging Effect on Epoxy-polysiloxane-Rice Husk Ash Polymeric Insulator Material
- Genetic Algorithm of Sliding Mode Control Design for Manipulator Robot
- Vision Based Self Localization for Humanoid Robot Soccer
- An Improved Robot Path Planning Algorithm
- Teleautonomous Control on Rescue Robot Prototype
- Indoor Localization System based on Artificial Landmarks and Monocular Vision
- Realistic Human Motion Preservation-Imitation Development on Robot with Kinect