SIMILARITY BASED ENTROPY ON FEATURE SELECTION FOR HIGH DIMENSIONAL DATA CLASSIFICATION
Abstract: Curse of
dimensionality is a major problem in most classification tasks. Feature
transformation and feature selection as a feature reduction method can be
applied to overcome this problem. Despite of its good performance, feature
transformation is not easily interpretable because the physical meaning of the
original features cannot be retrieved. On the other side, feature selection
with its simple computational process is able to reduce unwanted features and
visualize the data to facilitate data understanding. We propose a new feature
selection method using similarity based entropy to overcome the high
dimensional data problem. Using 6 datasets with high dimensional feature, we
have computed the similarity between feature vector and class vector. Then we
find the maximum similarity that can be used for calculating the entropy values
of each feature. The selected features are features that having higher entropy
than mean entropy of overall features. The fuzzy k-NN classifier was
implemented to evaluate the selected features. The experiment result shows that
proposed method is able to deal with high dimensional data problem with average
accuracy of 80.5%.
Author: Jayanti Yusmah Sari,
Mutmainnah Muchtar, Mohammad Zarkasi, Agus Zainal Arifin
Journal Code: jptkomputergg140016
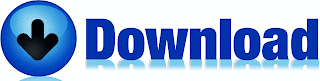