Development of a PSO-ANN Model for Rainfall-Runoff Response in Basins, Case Study: Karaj Basin
Abstract: Successful daily
river flow forecasting is necessary in water resources planning and management.
A reliable rainfall-runoff model can provide useful information for water
resources planning and management. In this study, particle swarm optimization
algorithm (PSO) as a metaheuristic approach is employed to train artificial
neural network (ANN). The proposed PSO-ANN model is applied to simulate the
rainfall runoff process in Karaj River for one and two days ahead. In this
regard, different combinations of the input variables including flow and
rainfall time series in previous days have been taken under consideration in
order to obtain the best model's performances. To evaluate efficiency of the
PSO algorithm in training ANNs, separate ANN models are developed using
Levenberg-Marquardt (LM) training algorithm and the results are compared with
those of the PSO-ANN models. The comparison reveals superiority of the PSO
algorithm than the LM algorithm in training the ANN models. The best model for
1 and 2 days ahead runoff forecasting has R2 of 0.88 and 0.78. Results of this
study shows that a reliable prediction of runoff in 1 and 2 days ahead can be
achieved using PSO-ANN model. Overall, results of this study revealed that an acceptable
prediction of the runoff up to two days ahead can be achieved by applying the
PSO-ANN model.
Author: Meysam Motahari, Hamed
Mazandaranizadeh
Journal Code: jptsipilgg170002
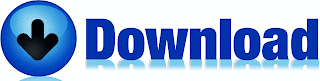