An Improved AP-Wishart Classifier for Polarimetric SAR Images by Incorporating a Textural Feature
Abstract: An improved
classifier is presented by imposing a textural feature to solve the problems of
vague initial clustering results, low classification accuracy and unchangeable
class number in the iterative classifier, based on H/Alpha decomposition and
the complex Wishart distribution for fully polarimetric SAR (Synthetic Aperture
Radar) images. First, wavelet transformation is used to extract texture from polarimetric
SAR images. Second, an AP (Affinity Propagation) algorithm is applied to create
the initial clustering result. This result is then applied to the iterative
classifier based on the complex Wishartdistribution to obtain the final result.
Two PALSAR (Phased Array type L-band Synthetic Aperture Radar) images from ALOS
(Advanced Land Observing Satellite) are used for the experiments carried out onexperimental
plots in Binhai Prefecture, Yancheng City, Jiangsu Province. The results show
that the improved classifier has some merits, including clear initial
clustering results, flexible class number and high classification accuracy. The
improved classifier has better overall performance than the original, and can be
effectively applied to the classification of polarimetric SAR images.
Author: Chen Jun
Journal Code: jptkomputergg150071
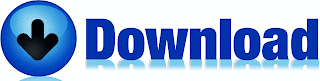