Computing Game and Learning State in Serious Game for Learning
Abstract: In order to support
the adaptive SGfL, teaching materials must be represented in game component
that becomes the target of adaptivity. If adaptive architecture of the game
only use game state (GS) to recognize player's state, SGfL require another
indicator -learning state (LS)- to identify the learning progress. It is a
necessary to formulate computational framework for both states in SGfL.The
computational framework was divided into two moduls, macro-strategy and
micro-strategy. Macro-strategy control the learning path based on learning map
in AND-OR Graph data stucture. This paper focus on the Macro-strategy modul,
that using online, direct, and centralized adaptivity method. The adaptivity in
game has five components as its target. Based on those targets, eight
development models of SGfL concept was enumerated. With similarity and
difference analysis toward possibility of united LS and GS in computational
framework to implement the nine SGfL concept into design and application, there
are three groups of the development models i.e. (1) better united GS and LS,
(2) must manage LS and GS as different entity, and (3) can choose whether to be
united or not. In the model which is united LS with GS, computing model at the
macro-strategy modul use and-or graph and forward chaining. However, in the
opposite case, macro-strategy requires two intelligent computing solutions,
those are and-or graph with forward chaining to manage LS collaborated with
Finite State Automata to manage GS. The proposed computational framework of
SGfL was resulted from the similarity and difference analysis toward all
possible representations of teaching materials into the adaptive components of
the game. It was not dependent of type of learning domain and also of the game
genre.
Author: Ririn Dwi Agustin, Ayu
Purwarianti, Kridanto Surendro, Iping S Suwardi
Journal Code: jptkomputergg150173
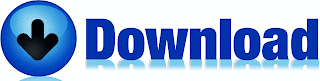
Artikel Terkait :
Jp Teknik Komputer gg 2015
- Current Ripple Analysis of New Double-Stator AC Drive Systems
- Comparing Performances of Evolutionary Algorithms on the Emission Dispatch and Economic Dispatch Problem
- A Polynomial-based Pairwise Key Pre-distribution and Node Authentication Protocol for Wireless Sensor Networks
- Power Characteristics of Wireless Charging for Sensor Networks in Tunnels
- Influence of Sensor Nodes on the Invulnerability of Tree Network
- An Automatic Calibration Method for Near-infrared Camera in Optical Surgical Navigation
- Optimal Economic Ordering Policy with Trade Credit and Discount Cash-Flow Approach
- Design and Implementation of Network Public Opinion Analysis System
- Evaluation of the Modernization of Hydraulic Projects Management Compact-Center-Point Triangular Whitenization Weight Function
- Transmission of Real-time Video Signal with Interference Density and Human Traffic
- Correlation of Student’s Precursor Emotion towards Learning Science Interest using EEG
- The Analysis of Rank Fusion Techniques to Improve Query Relevance
- Recognition of Emotions in Video Clips: The Self-Assessment Manikin Validation
- Automatically generation and evaluation of Stop words list for Chinese Patents
- Service Engineering Based on Service Oriented Architecture Methodology
- Modelling on Tracking Test Condition of Polymer Nanocomposite using Finite Element Simulation
- A Novel Approach to Optimize Cognitive Radio Network Utilization using Cascading Technique
- Classification of Non-Functional Requirements Using Semantic-FSKNN Based ISO/IEC 9126
- Poisson Clustering Process on Hotspot in Peatland Area in Sumatera
- Double Difference Motion Detection and Its Application for Madura Batik Virtual Fitting Room
- Alternative Technique Reducing Complexity of Maximum Attribute Relation
- High Performance Computing on Cluster and Multicore Architecture
- Control System for Nutrient Solution of Nutrient Film Technique Using Fuzzy Logic
- Measurement of Ultra Wideband Channel Sounding Using Vector Network Analyzer