Diagnostic Study Based on Wavelet Packet Entropy and Wear Loss of Support Vector Machine
Abstract: Against the
problems, the ratio of signal to noise of bearing wear is low, the feature
extraction is difficult, there are few fault samples and it is difficult to
establish the reliable fault recognition model, the diagnostic method is put
forward based on wavelet packet features and bearing wear loss of SVM. Firstly,
choose comentropy with strong fault tolerance as characteristic parameter, then
through wavelet packet decomposition, extract feature entropy of wavelet packet
in fault sensitivity band as input vector and finally, apply the Wrapper method
of least square SVM to choose optimal character subset. The application in
actual bearing fault diagnosis indicates the effectiveness of the proposed
method in the article.
Author: Yunjie Xu, Shudong Xiu
Journal Code: jptkomputergg140098
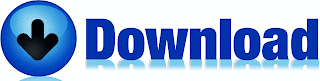