Face Recognition Using Invariance with a Single Training Sample
Abstract: For the limits of
memories and computing performance of current intelligent terminals, it is necessary
to develop some strategies which can keep the balance of the accuracy and
running time forface recognition. The purpose of the work in this paper is to
find the invariant features of facial images and represent each subject with
only one training sample for face recognition. We propose a two-layer hierarchical
model, called invariance model, and its corresponding algorithms to keep the
balance ofaccuracy, storage and running time. Especially, we take advantages of
wavelet transformations andinvariant moments to obtain the key features as well
as reduce dimensions of feature data based on thecognitive rules of human
brains. Furthermore, we improve usual pooling methods, e.g. max pooling and average
pooling, and propose the weighted pooling method to reduce dimensions with no
effect onaccuracy, which let storage requirement and recognition time greatly
decrease. The simulation results show that the proposed method does better than
some typical and nearly-proposed algorithms in balancing the accuracy and
running time.
Author: Qian Tian
Journal Code: jptkomputergg140131
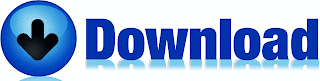
Artikel Terkait :
Jp Teknik Komputer dd 2014
- Development of Wireless Electric Field Mill for Atmospheric Electric Field Observation
- A Novel Intrusion Detection Approach using MultiKernel Functions
- Process Improvement of LSA for Semantic Relatedness Computing
- An Algorithm Based on Wavelet Neural Network for Garment Size Selection
- Dynamic DEMATEL Group Decision Approach Based on Intuitionistic Fuzzy Number
- A Reliable Web Services Selection Method for Concurrent Requests
- OWLS-CSM: A Service Profile Based Similarity Framework for Web Service Discovery
- Low Energy Adaptive Clustering Hierarchy Routing Protocol for Wireless Sensor Network
- Trusted Node-Based Algorithm to Secure Home Agent NATed IPv4 Network from IPv6 Routing Header Attacks
- Wireless Sensor Based Hybrid Architecture for Vehicular Ad hoc Networks
- Feature Selection Method Based on Improved Document Frequency
- Complex Optimization Problems Using Highly Efficient Particle Swarm Optimizer
- Image Fuzzy Enhancement Based on Self-Adaptive Bee Colony Algorithm
- Application of Chaotic Particle Swarm Optimization in Wavelet Neural Network
- Image Deblurring Via an Adaptive Dictionary Learning Strategy
- Multi-objective Optimization Based on Improved Differential Evolution Algorithm
- Unambiguous Acquisition for Galileo E1 OS Signal Based on Delay and Multiply
- Non-Planar MOSFET Modeling with Analytical Approach
- Pre-Timed and Coordinated Traffic Controller Systems Based on AVR Microcontroller
- Cooperative Avoidance Control-based Interval Fuzzy Kohonen Networks Algorithm in Simple Swarm Robots
- Windows Communication Foundation for Banyumas Tourism and Culinary Information System
- The use of ON-OFF and ANN Controllers for Automated Irrigation System Model Based on Penman-Monteith Evapotranspiration
- Cost Forecasting Model of Transmission Project based on the PSO-BP Method
- A New Algorithm for Detecting Local Community Based on Random Walk