Hierarchical Bayesian of ARMA Models Using Simulated Annealing Algorithm
Abstract: When the
Autoregressive Moving Average (ARMA) model is fitted with real data, the actual
value of the model order and the model parameter are often unknown. The goal of
this paper is to find an estimator for the model order and the model parameter
based on the data. In this paper, the model order identification and model
parameter estimation is given in a hierarchical Bayesian framework. In this
framework, the model order and model parameter are assumed to have prior
distribution, which summarizes all the information available about the process.
All the information about the characteristics of the model order and the model
parameter are expressed in the posterior distribution. Probability
determination of the model order and the model parameter requires the
integration of the posterior distribution resulting. It is an operation which
is very difficult to be solved analytically. Here the Simuated Annealing
Reversible Jump Markov Chain Monte Carlo (MCMC) algorithm was developed to
compute the required integration over the posterior distribution simulation.
Methods developed are evaluated in simulation studies in a number of set of
synthetic data and real data.
Author: S. Suparman, Michel
Doisy
Journal Code: jptkomputergg140029
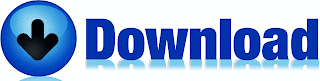
Artikel Terkait :
Jp Teknik Komputer gg 2014
- Research on Keyhole Diameter’s Vision Measurement Based on Parallel Technology
- Strain Transfer and Test Research of Stick-up Fiber Bragg Grating Sensors
- Matrix Mask Overlapping and Convolution Eight Directions for Blood Vessel Segmentation on Fundus Retinal Image
- Application Status of the Barkhausen Effect in Nondestructive Testing
- The New Complex-Valued Wavelet Neural Network
- A Review of Current Control Strategy for Single-Phase Grid-Connected Inverters
- Wireless Power Transfer by Using Solar Energy
- One-Time Password Implementation on Lego Mindstorms NXT
- Simple HAWT Prototype Efficiency at Small Scale Wind Speed
- Combine Target Extraction and Enhancement Methods to Fuse Infrared and LLL Images
- Batik Image Retrieval Based on Color Difference Histogram and Gray Level Co-Occurrence Matrix
- A Novel Part-of-Speech Set Developing Method for Statistical Machine Translation
- A Different Single-Phase Hybrid Five-Level Voltage-Source Inverter Using DC-Voltage Modules
- Multi Population Evolutionary Programming Approach for Distributed Generation Installation
- Failure Mechanism Analysis and Failure Number Prediction of Wind Turbine Blades
- Searching and Visualization of References in Research Documents
- Self-learning PID Control for X-Y NC Position Table with Uncertainty Base on Neural Network
- Adaptive Control of Space Robot Manipulators with Task Space Base on Neural Network
- Planar Finger-Shaped Antenna Used in Ultra-Wideband Wireless Systems
- Information Interchange Layer based on Classification of Information Use (IU)
- A Novel Multi-focus Image Fusion Method Based on Non-negative Matrix Factorization
- A Decision Tree Based on Spatial Relationships for Predicting Hotspots in Peatlands
- Traveling-Wave-Based Fault Location in Electrical Distribution Systems with Digital Simulations
- Multi-focus Image Fusion with Sparse Feature Based Pulse Coupled Neural Network
- Measuring Information Security Awareness of Indonesian Smartphone Users