Multi-focus Image Fusion with Sparse Feature Based Pulse Coupled Neural Network
Abstract: In order to better
extract the focused regions and effectively improve the quality of the fused image,
a novel multi-focus image fusion scheme withsparse feature basedpulse coupled
neural network (PCNN) is proposed. The registered source images are decomposed
into principal matrices and sparse matrices by robust principal component
analysis (RPCA).The salient features of the sparse matrices construct the
sparse feature space of the source images. The sparse features are used to
motivate the PCNN neurons. The focused regions of the source images are
detected by the output of the PCNN and integrated to construct the final fused
image. Experimental results showthat the proposed scheme works better in
extracting the focused regions and improving the fusion quality compared to the
other existing fusion methods in both spatial and transform domain.
Keywords: image fusion, robust
principal component analysis, pulse-coupled neural network, sparse feature,
firing times
Author: Yongxin Zhang, Li
Chen, Zhihua Zhao 1 , Jian Jia
Journal Code: jptkomputergg140065
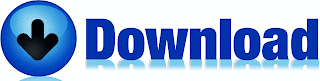