Ovarian Cancer Identification using One-Pass Clustering and k-Nearest Neighbors
Abstract: The identification
of ovarian cancer using protein expression profile (SELDI-TOF-MS) is important
to assists early detection of ovarian cancer. The chance to save patient’s life
is greater when ovarian cancer is detected at an early stage. However, the
analysis of protein expression profile is challenging because it has very high
dimensional features and noisy characteristic. In order to tackle those
difficulties, a novel ovarian cancer identification model is proposed in this
study. The model comprises of One-Pass Clustering and k-Nearest Neighbors
Classifier. With simple and efficient
computation, the performance of the model achieves Accuracy about 97%. This
result shows that the model is promising for Ovarian Cancer identification.
Author: Isye Arieshanti, Yudhi
Purwananto, Handayani Tjandrasa
Journal Code: jptkomputergg130112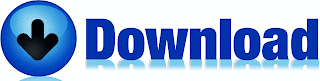
Artikel Terkait :
Jp Teknik Komputer gg 2013
- Gamelan Music Onset Detection based on Spectral Features
- Streamed Sampling on Dynamic data as Support for Classification Model
- A Mobile Ecotourism Recommendations System Using Cars-Context Aware Approaches
- Improved Harmony Search Algorithm with Chaos for Absolute Value Equation
- Future Smart Cooking Machine System Design
- A Review of Communication Protocols for Intelligent Remote Terminal Unit Development
- Power Balance AODV Algorithm of WSN in Agriculture Monitoring
- Circularly Polarized Proximity-Fed Microstrip Array Antenna for Micro Satellite
- Localizing Region-Based Level-set Contouring for Common Carotid Artery in Ultrasonography
- Separability Filter for Localizing Abnormal Pupil: Identification of Input Image
- Feature Extraction of Composite Damage on Acoustic Emission Signals
- A Camera Self-Calibration Method Based on Plane Lattice and Orthogonality
- Application of Wavelet Analysis in Detecting Runway Foreign Object Debris
- Palmprint Verification Using Time Series Method
- Time Series Based for Online Signature Verification
- Two-phase Flow Visualization Employing Gauss-Newton Method in Microchannel
- Study on Thermal Conductivity Methane Sensor Constant Temperature Detection Method
- Fuzzy Adaptive PID Control of a New Hydraulic Erecting Mechanism
- Optimization of Membership Functions for the Fuzzy Controllers of the Water Tank and Inverted Pendulum with Differents PSO Variants
- Study of an Improved Fuzzy Direct Torque Control of Induction Motor
- Research of NiMH Battery Modeling and Simulation Based on Linear Regression Analysis Method
- Design and Modeling of an Integrated Micro-Transformer in a Flyback Converter
- Renewable Distributed Generation Models in Three-Phase Load Flow Analysis for Smart Grid
- A Design Study of Dual-Stator Permanent Magnet Brushless DC Motor