A Hybrid Chebyshev-ICA Image Fusion Method Based on Regional Saliency
Abstract: An image fusion
method that performs robustly for image sets heavily corrupted by noise is presented
in this paper. The approach combines the advantages of two state-of-the-art
fusion techniques, namely Independent Component Analysis (ICA) and Chebyshev
Poly-nomial Analysis (CPA) fusion. Fusion using ICA performs well in
transferring the salient features of the input images into the composite output,
but its performance deteriorates severely under mild to moderate noise
conditions. CPA fusion is robust under severe noise conditions, but eliminates
the high frequency information of the images involved. We pro-pose to use ICA
fusion within high activity image areas, identified by edges and strong textured
surfaces and CPA fusion in low activity areas identified by uniform background
regions and weak texture. A binary image map is used for selecting the appropriate
method, which is constructed by a standard edge detector followed by
morphological operators. The results of the proposed approach are very
encouraging as far as joint fusion and denoising is concerned. The works
presented may prove beneficial for future image fusion tasks in real world
applications such as surveillance, where noise is heavily present.
Keywords: Image and data
fusion, Chebyshev polynomials, independent component analysis, regionbased
fusion
Author: Zaid Omar, Tania
Stathaki, Musa M. Mokji, Lila Iznita Izhar
Journal Code: jptkomputergg170061
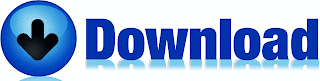