Forecasting Range Volatility using Support Vector Machines with Improved PSO Algorithms
Abstract: Financial volatility
forecasting is an important content in financial derivatives pricing, financial
riskmanagement, portfolio allocation. Support vector machines (SVM) has been successfully
used in modeling and forecasting volatility. To enhance the volatility
forecasting ability of SVM, this paper introduced three improved particle swarm
optimization (IPSO) algorithms into SVM for forecasting range volatility. SVM
was used to construct the volatility forecasting model. And, the three IPSO
algorithms: stochastic inertia weight PSO (SIWPSO), time-varying acceleration
coefficients PSO (TVACPSO) and two-order oscillating particle swarm
optimization (TOOPSO) were applied to finding the optimal parameters in SVM,
respectively. The accuracy of the proposed models in forecasting range
volatility was demonstrated by using three stock indices in China stock market.
The empirical results imply that compared to SVM with SIWPSO and TVACPSO algorithms,
SVM with TOOPSO algorithm produces higher accuracy in forecasting range volatility
and faster speed on searching for the optimal parameters of SVM.
Keywords: volatility
forecasting, support vector machines, improved particle swarm optimization algorithm
Author: Liyan Geng
Journal Code: jptkomputergg160075
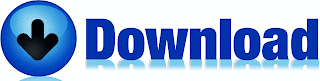