Low Complexity Sparse Channel Estimation Based on Compressed Sensing
Abstract: In wireless
communication, channel estimation is a key technology to receive signal
precisely. Recently, a new method named compressed sensing (CS) has been proposed
to estimate sparse channel, which greatly improves the spectrum efficiency.
However, it is difficult to realize it due to its high computational
complexity. Although the proposed Orthogonal Matching Pursuit (OMP) can reduce
the complexity of CS, the efficiency of OMP is still low because only one index
is identified per iteration. Therefore, to solve this problem, more efficient
schemes are proposed. At first, we apply Generalized Orthogonal Matching
Pursuit (GOMP) to channel estimation, which lower computational complexity byselecting
multiple indices in each iteration. Then a more effective scheme that selects
indices by leastsquares (LS) method is proposed to significantly reduce the
computational complexity, which is a modifiedmethod of GOMP. Simulation results
and theoretical analysis show the effectivity of the proposed algorithms.
Author: Fei Zhou, Yantao Su,
Xinyue Fan
Journal Code: jptkomputergg160257
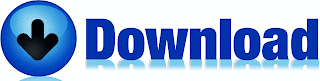