Multi-Criteria in Discriminant Analysis to Find the Dominant Features
Abstract: A crucial problem in
biometrics is enormous dimensionality. It will have an impact on the costs involved.
Therefore, the feature extraction plays a significant role in biometrics
computational. In thisresearch, a novel approach to extract the features is
proposed for facial image recognition. Four criteria of the Discriminant
Analysis have been modeled to find the dominant features. For each criterion is
an objective function, it was derived to obtain the optimum values. The optimum
values can be solved by using generalized the Eigenvalue problem associated to
the largest Eigenvalue. The modeling results were employed to recognize the
facial image by the multi-criteria projection to the original data. The
training sets were also processed by using the Eigenface projection to avoid
the singularity problem cases. The similarity measurements were performed by
using four different methods, i.e. Euclidian Distance,Manhattan, Chebyshev, and
Canberra. Feature extraction and analysis results using multi-criteria have shown
better results than the other appearance method, i.e. Eigenface (PCA),
Fisherface (Linear Discriminant Analysis or LDA), Laplacianfaces (Locality
Preserving Projection or LPP), and Orthogonal Laplacianfaces (Orthogonal
Locality Preserving Projection or O-LPP).
Keywords: multi-criteria,
discriminant analysis, features extraction, singularity problem, facial
recognition
Author: Arif Muntasa, Indah
Agustien Siradjuddin, Rima Tri Wahyuningrum
Journal Code: jptkomputergg160310
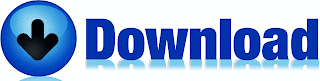
Artikel Terkait :
Jp Teknik Komputer gg 2016
- An Improved Artificial Bee Colony Algorithm for Staged Search
- A Novel Multifunction Digital Chip Design Based on CMOS Technology
- An Improved Adaptive Niche Differential Evolution Algorithm
- Power Quality Analysis of Integration Photovoltaic Generator to Three Phase Grid under Variable Solar Irradiance Level
- A Combined User-order and Chunk-order Algorithm to Minimize the Average BER for Chunk Allocation in SCFDMA Systems
- An Optimized Model for MapReduce Based on Hadoop
- Hierarchical i* Modeling in Requirement Engineering
- The Optimal High Performance Computing Infrastructure for Solving High Complexity Problem
- Transformer Fault Diagnosis Method Based on Dynamic Weighted Combination Model
- Hybrid Hierarchical Collision Detection Based on Data Reuse
- Brightness and Contrast Modification in Ultrasonography Images Using Edge Detection Results
- Recognition of Fission Signals Based on Wavelet Analysis and Neural Network
- Application of Nonlinear Dynamical Methods for Arc Welding Quality Monitoring
- Chaos-Enhanced Cuckoo Search for Economic Dispatch with Valve Point Effects
- GPU CUDA Accelerated Image Inpainting using Fourth Order PDE Equation
- Comparative Analysis of Spatial Decision Tree Algorithms for Burned Area of Peatland in Rokan Hilir Riau
- Action Recognition of Human’s Lower Limbs Based on a Human Joint
- A Soft Error Study on Tri-gate Based FinFET and Junctionless-FinFET 6T SRAM Cell - A Comparison
- Design of AC Charging Interface and Status Acquisition Circuit for Electric Vehicles
- Towards Smooth and High-Quality Bitrate Adaptation for HTTP Adaptive Streaming
- Features Deletion on Multiple Objects Recognition
- Research on Batch Scheduling in Cloud Computing
- Classification of Motorcyclists not Wear Helmet on Digital Image with Backpropagation Neural Network
- Internet Protocol Based Satellite On-Board System