Predicting the Presence of Learning Motivation in Electronic Learning: a New Rules to Predict
Abstract: Research affirms
that electronic learning (e-learning) has a great deal of advantages to
learning process, it’s provides learners with flexibility in terms of time,
place, and pace. That easiness was not be a guarantee of a good learning
outcomes though they got the same learning material and course, because the
fact is the outcomes was not the same from one to another and even not satisfy
enough. One of prerequisite to the successful of e-learning process is the
presence of learning motivation and it was not easy to identify. We propose a
novel model to predicting the presence of learning motivation in e-learning
using those attributes that have been identified in previous research. This
model has been built using WEKA toolkit by comparing fourteen algorithms for
Tree Classifier and ten-fold cross validation testing methods to process 3.200
of data sets. The best accuracy reached at 91.1% and identified four parameters
to predict the presence of learning motivation in e-learning, and aim to assist
teachers in identify whether student needs motivation or does not. This study
also confirmed that Tree Classifier still has the best accuracy to predict and
classify academic performance, it was reached average 90.48% for fourteen
algorithm for accuracy value.
Author: Christina Juliane,
Arry A. Arman, Husni S. Sastramihardja, Iping Supriana
Journal Code: jptkomputergg170133
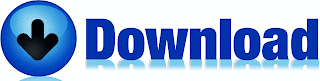