Regression Modelling for Precipitation Prediction Using Genetic Algorithms
Abstract: This paper discusses
the formation of an appropriate regression model in precipitation prediction. Precipitation
prediction has a major influence to multiply the agricultural production of
potatoes in Tengger, East Java, Indonesia. Periodically, the precipitation has
non-linear patterns. By using a non-linear approach, the prediction of
precipitation produces more accurate results. Genetic algorithm (GA) functioning
chooses precipitation period which forms the best model. To prevent early
convergence, testing the best combination value of crossover rate and mutation
rate is done. To test the accuracy of the predicted results are used Root Mean
Square Error (RMSE) as a benchmark. Based on the RMSE value of each method on
every location, prediction using GA-Non-Linear Regression is better than Fuzzy Tsukamoto
for each location. Compared to Generalized Space-Time Autoregressive-Seemingly
Unrelated Regression (GSTAR-SUR), precipitation prediction using GA is better.
This has been proved that for 3 locations GA is superior and on 1 location, GA
has the least value of deviation level.
Author: Asyrofa Rahmi, Wayan
Firdaus Mahmudy
Journal Code: jptkomputergg170122
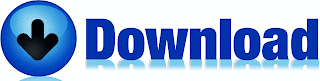