Sparse Coding-Based Method Comparison For Land-Use Classification
Abstract: Land-use
classification utilize high-resolution
remote sensing image. The image is utilized for improving the classification
problem. Nonetheless, in other side, the problem becomes more challenging cause
the image is too complex. We have to represent the image appropriately. On of
the common method to deal with it is Bag of Visual Word (BOVW). The method needs a coding process to get the final data
interpretation. There are many methods to do coding such as Hard Quantization
Coding (HQ), Sparse Coding (SC), and Locality-constrained Linear Coding (LCC).
However, that coding methods use a different assumption. Therefore, we have to
compare the result of each coding method. The coding method affects
classification accuracy. The best coding method will produce the better
classification result. Dataset UC Merced consisted 21 classes is used in this
research. The experiment result shows that LCC got better performance /
accuracy than SC and HQ. LCC method got 86.48 % accuracy. Furthermore, LCC also
got the best performance on various number of training data for each class.
Keywords: Land-use
classification; high-resolution remote sensing image; Bag of Visual Word
(BOVW); Sparse Coding (SC); Hard Quantization Coding (HQ)
Author: Dewa Made Sri Arsa,
Grafika Jati, M H Hilman
Journal Code: jptkomputergg170013
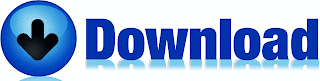