Meta-Algoritme Adaptive Boosting untuk Meningkatkan Kinerja Metode Klasifikasi pada Prestasi Belajar Mahasiswa
Abstract: Determining the
right class on student achievement is important in an evaluation process,
because placing students in the right class helps lecturer in reflecting the
successfullness of learning process. This problem relates to the performance of
classification method which is measured by the classifier metrics. High
performance is indicated by the optimality of these classifier's metrics.
Besides, meta-algorithm adaptive boosting has been proven to be able to improve
the performance of classifier in various fields. Therefore, this paper employs
adaptive boosting to reduce the number of incorrect student placement in a
class. The experimental results of implementing adaptive boosting in
classifying student achievement shows that there is an increase of performance
of all classification metrics, i.e., Kappa, Precision, Recall, F-Measure, ROC,
and MAE. In terms of accuracy, J-48 is able to rise about 3.09%, which means
this method reduces three misclassified students. Additionally, decisionStump
increases 12.37% of accuracy. This also means this method is able to decrease
12 misclassified students. Finally, Simple Cart reaches the highest accuracy of
about 23.71%, while the number of misclassified students is reduced to 24
students. However, there is no improvement in Random Forest method by using
this adaptive boosting.
Penulis: Yuni Yamasari, Supeno
M. S. Nugroho, Dwi F. Suyatno, Mauridhi H. Purnomo
Kode Jurnal: jptlisetrodd170496
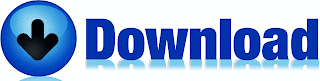